The disadvantages of large language models
Medical Pharmaceutical Translations • Aug 7, 2023 12:00:00 AM
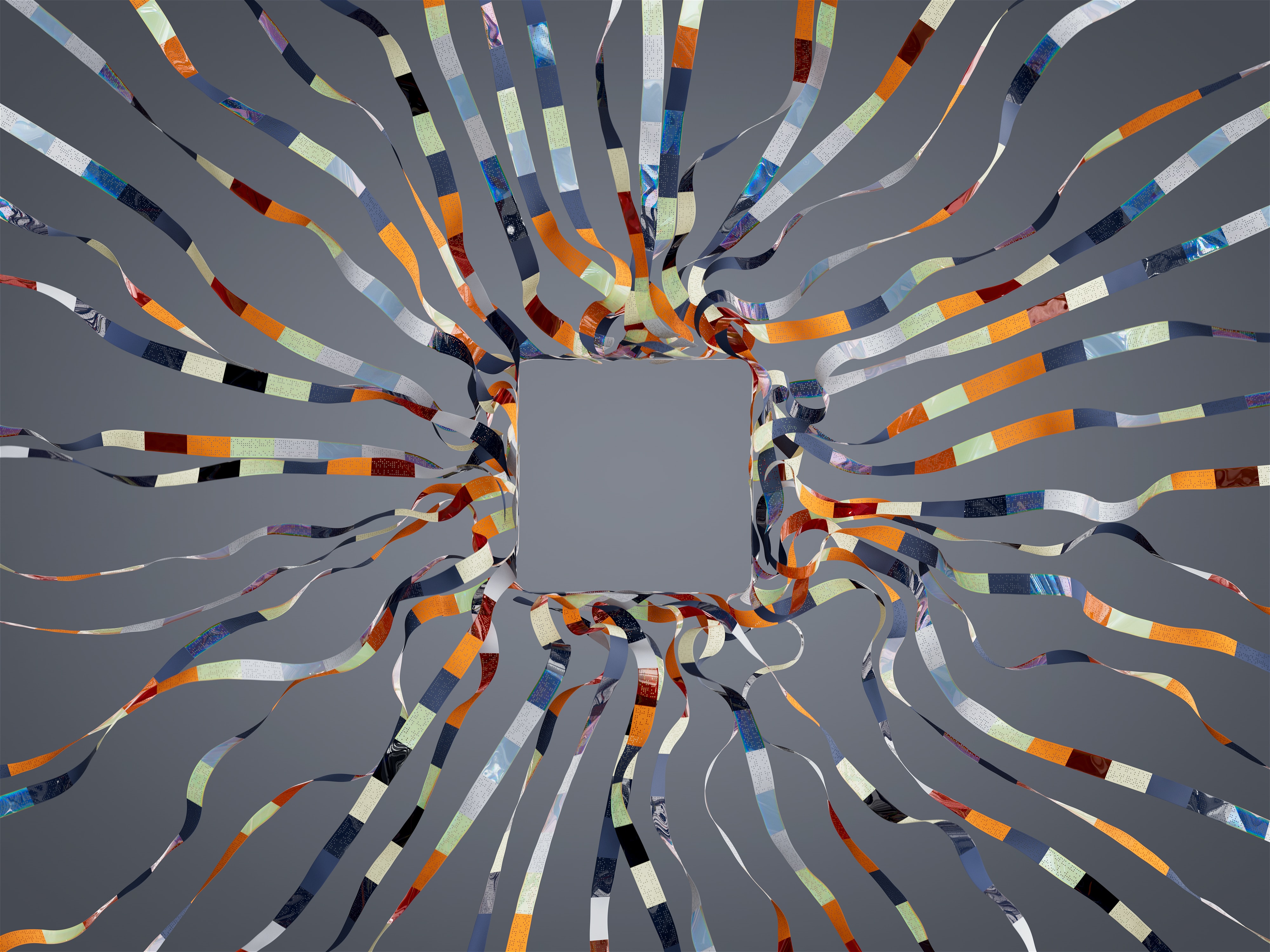
If you’re on the internet a lot, or if you’ve used a customer service helpline or chat recently, chances are you’ve dealt with content generated by a large language model.
Large language models are essentially algorithms that analyze enormous quantities of text in order to recognize patterns that will allow them to imitate human speech and writing. The best-known example of a large language model is the famous ChatGPT.
Large language models’ output can be impressive. In many cases, for instance, you may not even realize you’re reading text or a translation generated by a machine. (For the record, I promise I’m a real person, not a robot). This means that AI can now be used to do tasks like provide customer service help; create documents, articles and translations; and imitate human speech, among other things.
But while they can be genuinely helpful, large language models also come with some important disadvantages, many of which are downright troubling.
In a recent article, journalist Donovan Johnson shared some of large language models’ most significant downsides.
These include:
● lack of privacy protection. “Privacy,” Johnson writes, “is a primary concern surrounding AI language models.” He explains that the vast pool of sources language learning models like ChatGPT use includes social media platforms and even personal websites. In some cases, internet users’ personal data isn’t used without consent. For instance, the European Union’s General Data Protection Regulation (GDPR) requires people to give explicit consent to use any information they might share online (usually by clicking on a pop-up window before accessing a website or social media platform). But laws like these don’t exist everywhere, and even then, users may not be fully aware of the implications of what they’re consenting to.
For instance….
● ignoring “the right to be forgotten”. Johnson points out that just because internet users might delete posts and information, if it’s already been studied by a large language model, this information will still be a part of its pool of data. Developers are working to find a way for information like this to be deleted, but so far there’s no guaranteed, standard way for anyone to entirely delete their information or request that it be deleted.
● questionable HIPAA compliance. Those familiar with healthcare regulations may be wondering about the implication large language models’ challenge to privacy has on HIPAA compliance. As we’ve reported before, for some experts, most large language models, including ChatGPT, are not HIPAA compliant. There are some workarounds and precautions that individual users and organizations can implement to protect sensitive information, but these solutions aren’t accessible to everyone.
● potential inaccuracies. Almost all of us have probably experienced a chatbot making a mistake. For instance, you’ve probably been frustrated at least once when using a customer service ‘bot that just couldn’t understand what you needed or gave you an incorrect or unhelpful response. These errors are based on the fact that language models’ pool of data and understanding of the subtleties of language are limited. This problem extends to just about all of large language models’ uses. That’s one of the many reasons why anyone in charge of sharing important information should think twice before relying on machines alone.
While these are already significant disadvantages, they’re not the only ones. Other journalists and experts have cited additional problems with large language models, including:
● environmental impact. Large language models require a lot of energy to operate, which means they’re having a bad impact on the environment.
● a limited pool of data. Several sources, including this helpful explainer, reveal that some large language models become overly dependent on the pool of data they’ve analyzed, and are unable to integrate new, updated, or additional information. Tech expert Becky Abraham adds that this also means large language models can’t access websites or other sources that they haven’t been programmed to use.
These factors make AI-generated news articles, health information, and certain research tools less reliable.
● possible built-in errors. Abraham writes that a large language model can be improperly programmed, or that it could recognize errors, misinformation, or outdated facts as accurate and not have these mistakes corrected by its programmers.
● bias. Large language models analyze available data, but because they’re not human, they can’t always recognize hate speech or seek out diversity. This has led to a number of issues, from chatbots learning hate speech to biases in gender, age, race, and other features when it comes to things like image and information searches. Although their name would suggest the opposite, large language models are even biased when it comes to…language. We’ll look more deeply into the issue of bias in large language models in next week’s article.
Large language models have proven incredibly useful in a number of ways. But as this list shows, nobody’s perfect, not even a robot. Some tasks still require a human touch, or at least human help.
In many cases, monitoring or editing by humans would help reduce the errors, biases, and privacy issues that are currently an inherent part of working with large language models. Whenever possible, relying on humans to write articles, translate texts and speech, and help customers and patients is still the best way to ensure that communications are clear, accurate, and privacy compliant.
Contact Our Writer – Alysa Salzberg