Why language models are biased - and what that means for those who use them
Medical Pharmaceutical Translations • Aug 14, 2023 12:00:00 AM
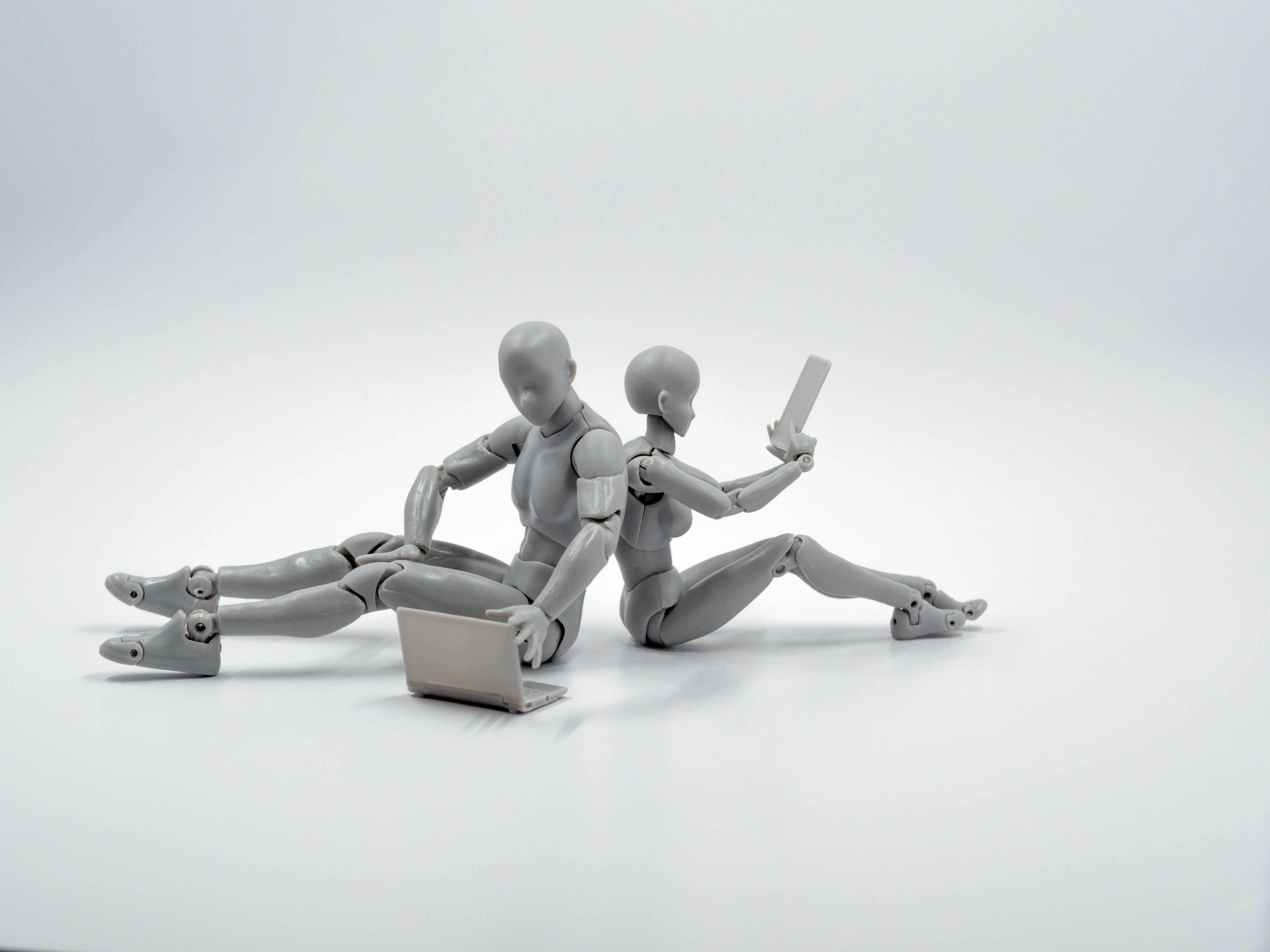
Large language models are algorithms that analyze vast amounts of text and other sources in order to “learn” how to imitate human speech and writing. Many large language models, like the famous ChatGPT, can often accomplish this to an impressive degree (or so it might seem). But like just about anything in life, large language models have their disadvantages.
One of the most troubling of these is bias. Experts and laypersons who’ve used or interacted with a large language model (for instance, in the form of a chatbot or translation AI), have noticed that this AI shows bias in a number of ways, including towards gender and even language.
These biases exist because AI is evil; they’re due to the fact that large language models learn from what’s given to them.
It’s a lesson humans famously learned all the way back in 2016, when Microsoft proudly released Tay, a chatbot who would continue to learn by having conversations with real people, allowing it, ultimately, to seem like a real person in its interactions with participants. The only problem was, a number of participants decided to post racist and sexist comments, which the ‘bot understood as normal responses and integrated into its own speech patterns, tweeting them to the world. In the end, Microsoft’s promising AI ended up being a racist, sexist mess…and a terrible reflection on humanity.
Still, that hasn’t stopped programmers from continuing to develop large language models - and in many ways, this is a good thing, since there are a number of useful applications for them. Still, even if programmers can avoid errors like allowing people to directly “teach” AI racist and sexist comments, they can’t entirely avoid certain biases.
For instance, a few years ago we took a look at Google Translate’s gender bias.
Like Tay’s racism and sexism, this bias comes from what Google Translate’s AI had to learn from - a pool of millions of online documents, many of which are books and other archival material that date back decades or even centuries. And so, for instance, while nowadays it shouldn’t automatically be assumed that a doctor is a man and a nurse is a woman, Google Translate was automatically assigning these genders to these professions, based on what its AI had learned.
One piece of good news: Google’s programmers have been able to generate translations that show a sentence with a male and a female option. But this isn’t yet possible in translations of longer texts.
Another way that large language models are biased is more subtle. In a recent interview on A19’s Techtonic podcast, researchers Aliya Bhatia and Gabriel Nicholas spoke about findings from their fascinating report on large and multilingual language models. One thing they shared is that language models favor English over other languages. This is because more than half of available content on the internet content is in English.
This means that when developers try to create multilingual language models, they have their work cut out for them.
Some of the major issues with multilingual language models, Bhatia and Nicholas report, include:
● highly specific, biased, and/or obsolete sources. While many languages might have a wide range of written material for AI to learn from, others are limited to highly specific sources, like religious or government texts. This means that translations into these languages won’t necessarily be an accurate representation of how they’re spoken in everyday life. Additionally, Bhatia and Nicholas point out that many of these limited sources may be biased - for instance, government communications may not use a lot of negative language, limiting AI’s options.
● sources may have been auto-translated. Interestingly, Bhatia and Nicholas reveal, for some languages with very few written sources, material that does exist may actually be AI-generated translations, meaning their accuracy is dubious, especially when it comes to capturing contemporary speech accurately.
● inability to localize. No matter how smart AI can be, it’s not capable of understanding the subtleties of language, including figurative language and double meanings. Bhatia and Nicolas give an example of the word uso in Basque. This word translates to “dove” in English, which would imply to AI programmed to seek out hate speech that it’s associated with peace. But in fact, in contemporary Basque, uso can be an insult that would fall under hate speech.
The issue becomes even more complicated when connotation - another aspect of language that AI can’t comprehend - is added to the mix. For instance, Bhatia and Nicholas write that the phrase “Bengali Muslim”, which is neutral in most cultures, is a hateful insult in the Assamese language, due to the historical and cultural context behind the phrase for Assamese speakers.
● challenges with multilingualism. Unlike human translators, large language models have a hard time understanding and making connections between multiple languages. Interestingly, the more languages you input into a multilingual language model, the less capable the AI is of correctly understanding and using each one. Bhatia and Nicholas report that many programmers have to prioritize which language or languages will be the one(s) their multilingual model knows best. This can have a number of consequences, including the prioritizing of languages tied to wealthy populations as opposed to poor ones.
Fortunately, there are some possible ways to fix these problems. But as with the attempt to remove Google Translate’s gender bias, the solutions suggested by Bhatia and Nicholas can’t be implemented overnight. Many could take a long time, or even be impossible to implement under certain circumstances.
For now, one of the best ways to deal with large language and multilingual language models is to be aware that despite how exciting they are and how far AI has come, they can’t be solely relied on for things like accurate translations, especially for less common languages.
We’re often told that robots are smarter than humans. But in many cases, when emotions and critical thinking are needed, language models fall short. AI like ChatGPT and Google Translate are good in a pinch, but truly successful translations require more than just knowing word patterns. They have to reflect how people really speak a language, and rely on localization as much as translation. In other words, no matter how far technology has come, a truly still good translation needs a human touch.
Contact Our Writer – Alysa Salzberg